Artificial intelligence (AI) is rapidly becoming integrated into our lives, from virtual assistants to complex diagnostic tools. As we rely on these systems more, a crucial question arises: how do we trust them? Interestingly, our approach to trusting AI often differs dramatically from how we trust humans, especially those still learning.
Consider this simple, hand-drawn graph:
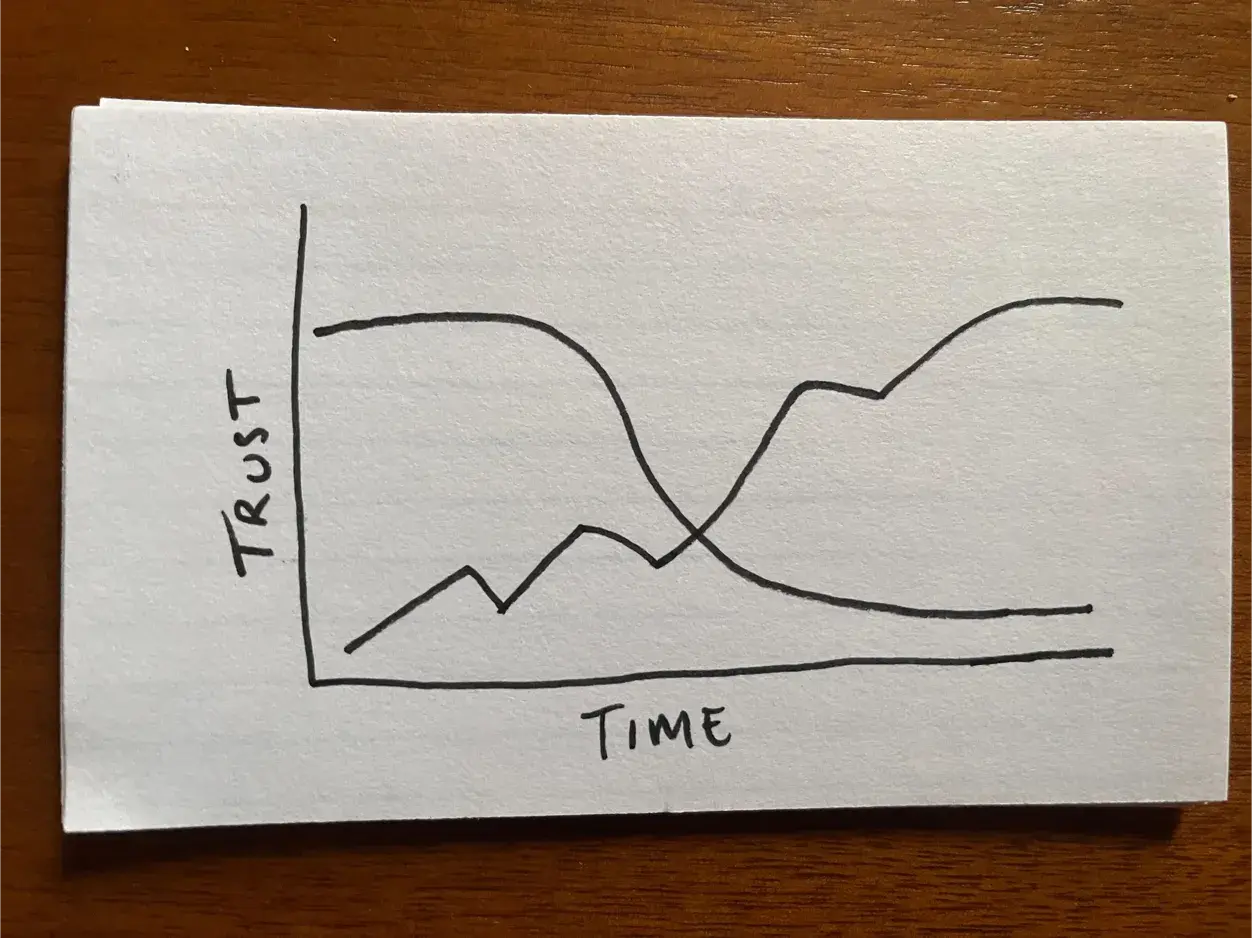
The X-axis represents time, and the Y-axis represents the level of trust. It depicts two distinct journeys, highlighting a fascinating aspect of human psychology when interacting with technology versus fellow humans. Let's break it down.
The AI trust paradox: Starting high, falling hard
The top line on the graph represents a common trajectory for trust in a new AI system. Notice how it starts very high?
- Borrowed trust: We often grant AI systems significant initial trust. Why? Because we have decades of experience with traditional software that is largely deterministic and reliable. Calculators don't suddenly get math wrong; operating systems (usually!) perform core functions predictably. We subconsciously project this expectation of near-perfect reliability onto sophisticated AI.
- The unforgiving fall: Look at that sharp, early drop. This illustrates what happens when the AI makes a mistake. Because our initial expectation was so high – bordering on perfection – any error feels like a significant betrayal. It's not just a mistake; it feels like a fundamental flaw in the system. This violation of expectation leads to a dramatic and often disproportionate loss of trust. We are, as the initial prompt suggested, remarkably unforgiving.
- Fragile recovery: Rebuilding that trust is a struggle, as shown by the volatile path afterward. Subsequent errors, even minor ones, can reinforce the perception of unreliability, making it difficult for the AI to regain our full confidence. The "black box" nature of some AI systems doesn't help – if we don't understand why it failed, it's harder to trust it won't fail again.
The human learner curve vs AI: Earning trust through growth
Now look at the bottom line, representing how we might build trust in, say, a junior employee or an apprentice.
- Starting low, expectations managed: Trust begins at a much lower level. We know the person is inexperienced. We hire or mentor them with the explicit understanding that they are learning and mistakes are part of the process. Our initial expectations are calibrated accordingly.
- Building through experience: The line shows a gradual upward trend. As the employee listens, learns, successfully completes tasks, demonstrates improvement, and responds to feedback, our trust grows. It's earned incrementally over time through observed competence and effort.
- Mistakes as learning opportunities: Notice the small dips? These represent mistakes. However, unlike the catastrophic drop for the AI, these errors don't usually destroy trust. Provided they are acknowledged, learned from, and not indicative of negligence, we tend to view them as part of the expected learning curve. We offer feedback, guidance, and forgiveness, often strengthening the relationship (and trust) if the employee responds well.
Why the stark difference between expectations for AI vs people?
The contrast between these two lines boils down to fundamental differences in our approach:
- Initial expectations: We expect near-perfection from technology, including AI, but expect learning and imperfection from novice humans.
- Error tolerance: Our tolerance for AI errors is incredibly low due to high initial expectations. Our tolerance for human error (especially from learners) is higher, assuming it leads to growth.
- Perceived nature: We see AI as a tool that should work flawlessly. We see an employee as a person with potential, fallibility, and the capacity to learn and improve through interaction.
- Feedback and understanding: We can talk to the human learner, understand their reasoning (or lack thereof), provide direct feedback, and observe their response. This communication loop is vital for building trust but is often missing or opaque with AI.
Changing the way we view trust in AI
Understanding the difference in expectations between AI and people is crucial as AI becomes more prevalent. We need to shift from expecting deterministic perfection to understanding AI as a probabilistic tool with limitations – powerful, but not infallible. Managing our expectations, demanding transparency in AI systems, and developing better ways to understand and interact with AI when things do go wrong will be key to building more realistic and resilient trust in the technologies shaping our future.
Perhaps we need to learn to grant AI something akin to the "learner's permit" we grant our human colleagues – acknowledging its capabilities while being prepared for, and constructive about, its inevitable mistakes.
Cutover: AI with human oversight for IT operations
So how do we bridge this trust gap, moving away from brittle and potentially over-hyped expectations of AI towards a more developmental model akin to how we nurture human expertise? We at Cutover are addressing this head-on, particularly with Agentic AI operating within Cutover runbooks allowing for the interconnection between human and machine tasks, which lets us all gain this crucial trust.
Cutover makes it straightforward to implement the checks and balances—both before an AI agent executes and after its completion—teams can then confidently verify the data an agent has used and validate its outcome. This human oversight ensures clarity and control before proceeding to the next step and helps teams evolve trust in AI capabilities, much like we cultivate with a human expert.
Learn how Cutover is helping technology operations teams with AI-powered runbooks at cutover.ai.